AI on a Budget: Strategies for Cost-Effective Implementation in Modern Enterprises
Discover cost-effective AI implementation strategies to reduce AI development costs while maximizing ROI. Explore budget-friendly tools, outsourcing, and optimization techniques.
2025-03-21 06:58:28 - techhive-nextgen
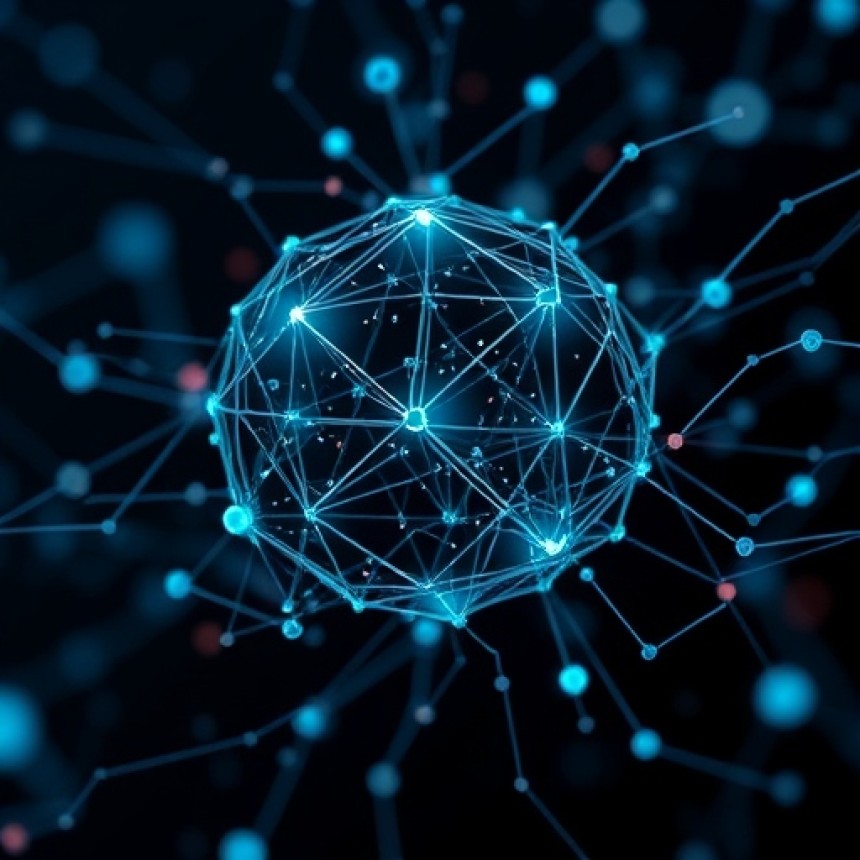
Introduction
Artificial Intelligence (AI) has become an integral part of modern enterprises, driving automation, enhancing decision-making, and improving customer experiences. However, one of the biggest challenges businesses face is the AI development cost. Implementing AI solutions can be expensive, particularly for small and medium-sized enterprises (SMEs) that may lack the resources of tech giants.
This article explores the various factors influencing AI development costs and offers cost-effective strategies for enterprises looking to implement AI without breaking the bank.
AI development costs vary depending on several factors, including:
- Type of AI Solution: Custom-built AI models are more expensive than off-the-shelf solutions.
- Data Collection and Processing: AI relies on vast amounts of data, and acquiring high-quality datasets can be costly.
- Infrastructure and Hardware: On-premise AI requires high-performance computing resources.
- Talent and Development: Hiring data scientists, AI engineers, and machine learning experts adds to costs.
- Integration and Maintenance: Ensuring seamless AI integration into existing systems requires additional resources.
Using open-source AI frameworks like TensorFlow, PyTorch, and Scikit-learn can significantly reduce AI development costs. These platforms provide pre-built models and tools that enable businesses to develop AI solutions without licensing fees.
Cloud-based AI solutions offer scalability and cost efficiency compared to on-premise infrastructure. Providers like Google AI, Amazon SageMaker, and Microsoft Azure AI provide AI-as-a-Service (AIaaS) models, allowing businesses to pay only for what they use.
Low-code and no-code AI platforms enable businesses to build AI applications with minimal programming expertise. Tools like DataRobot, Google AutoML, and Microsoft Power Automate can help companies deploy AI without the need for a dedicated AI development team.
Instead of building an in-house AI team, outsourcing AI development to experienced service providers can be more cost-effective. Offshore AI development companies offer competitive pricing while delivering high-quality AI solutions.
Pre-trained AI models can reduce development time and costs. Platforms like Hugging Face, IBM Watson, and OpenAI provide pre-trained models for natural language processing (NLP), image recognition, and predictive analytics.
Optimizing infrastructure helps in cutting costs. Businesses can:
- Use serverless computing to run AI workloads without dedicated servers.
- Implement AI model compression to reduce computational requirements.
- Utilize batch processing instead of real-time processing to lower costs.
To ensure a good return on investment (ROI), businesses should:
- Align AI with business goals to maximize its impact.
- Continuously monitor and optimize AI models to improve efficiency.
- Measure key performance indicators (KPIs) to track AI success and cost-effectiveness.
A mid-sized e-commerce company implemented AI chatbots using pre-trained NLP models, reducing customer support costs by 40% while improving response times.
Case Study 2: Predictive Maintenance in ManufacturingA manufacturing firm leveraged cloud-based AI services to predict machine failures, saving thousands of dollars in unplanned downtime costs.
Advancements in no-code AI platforms will make AI more accessible to non-technical users, further reducing development costs.
2. AI-Powered Automation ToolsAI-driven automation will streamline repetitive business processes, cutting down operational expenses.
3. AI Cost Optimization SolutionsNew AI tools focused on cost optimization will help businesses monitor and control AI development costs in real-time.
AI development doesn’t have to be prohibitively expensive. By leveraging open-source tools, cloud services, and pre-trained models, enterprises can implement AI efficiently while keeping costs under control. Strategic outsourcing and infrastructure optimization further enhance affordability, ensuring businesses maximize their ROI from AI investments.
AI development costs vary based on project complexity. Simple AI models can cost $10,000 - $50,000, while advanced enterprise AI solutions may range from $100,000 to $500,000 or more.
Small businesses can reduce AI costs by using low-code AI platforms, pre-trained models, and cloud-based AI services, avoiding the need for expensive in-house teams.
Hidden costs include data acquisition, model training, cloud storage fees, integration with existing systems, and ongoing maintenance.
Yes, outsourcing AI development can be cost-effective, especially when working with experienced AI development companies that offer competitive pricing.
To optimize costs, businesses can choose scalable AI solutions, implement AI model compression techniques, and leverage cloud-based AI services instead of on-premise infrastructure.
Some of the best open-source AI tools include TensorFlow, PyTorch, Scikit-learn, Hugging Face, and OpenAI GPT models, which provide free and powerful AI capabilities.